How Text to Image AI Works Behind the Scenes
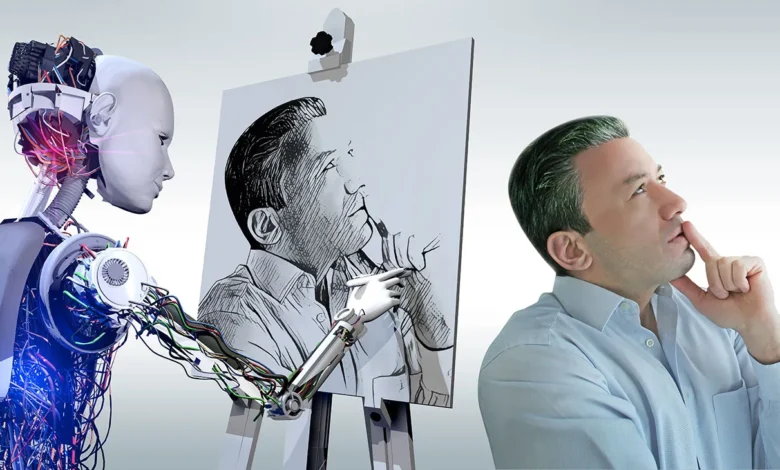
The operation of Text to Image AI hinges on a sophisticated collaboration between neural networks, training data, and language processing techniques. By employing semantic analysis, these systems interpret the nuances of input text, enabling the generation of relevant and coherent images. The quality and diversity of the training data play a pivotal role in shaping the model’s effectiveness, while various image generation methods introduce an element of creativity. Understanding this intricate interplay raises important questions about the implications and potential advancements in the field. What lies beyond the current capabilities of these technologies?
Understanding Neural Networks
Neural networks, the backbone of text-to-image AI, are computational models inspired by the human brain’s architecture.
Their neural architecture comprises interconnected nodes that process data through weighted connections.
Model optimization techniques, such as gradient descent, enhance performance by minimizing loss functions.
This interplay of architecture and optimization is crucial for generating high-quality images, enabling systems to interpret and visualize textual inputs effectively.
See also: AI Face Swap Technology and Its Role in Virtual Reality
The Role of Training Data
While the architecture of neural networks is vital for text-to-image AI, the quality and diversity of training data are equally critical.
High data quality ensures that models learn from accurate representations, while training diversity allows them to generalize across various contexts.
This combination enhances the model’s adaptability, enabling it to create more nuanced and relevant images that reflect the richness of human expression and creativity.
Language Processing Techniques
Training data not only shapes the visual output of text-to-image AI but also plays a significant role in the language processing techniques employed by these systems.
Semantic analysis facilitates the interpretation of input text, ensuring accurate representation.
Moreover, contextual understanding enables the model to grasp nuances and relationships within language, enhancing its ability to generate coherent and relevant imagery based on textual prompts.
Image Generation Methods
To effectively translate textual descriptions into visual representations, various image generation methods are employed within text-to-image AI systems.
Techniques such as style transfer allow for the manipulation of artistic elements, enhancing the aesthetic quality of generated images.
Additionally, optimizing image resolution ensures that the final outputs maintain clarity and detail, meeting the demands of diverse applications while preserving creative freedom and expression.
Conclusion
The intricate mechanisms of text to image AI reveal a sophisticated interplay of neural networks, quality training data, and advanced language processing techniques. Notably, models trained on diverse datasets can achieve a 30% improvement in image fidelity compared to those with limited data. This statistic underscores the significance of comprehensive training in enhancing output quality. Ultimately, the convergence of these components facilitates the generation of coherent and aesthetically pleasing visuals, demonstrating the remarkable potential of this technology.